The financial sector is rapidly adopting advanced technologies to elevate customer experience and optimize operational efficiency. Digital twins have become pivotal, not only for gaining deeper customer insights but also for enabling innovative solutions across diverse fintech challenges. At the forefront of this transformation stands Twinize, a comprehensive digital twin platform specifically designed for the financial sector that empowers banks to realize the full potential of their digital transformation journey. In this context, it's valuable to revisit what digital twins are and examine their transformative potential within the banking sector and how Twinize helps this transformation.
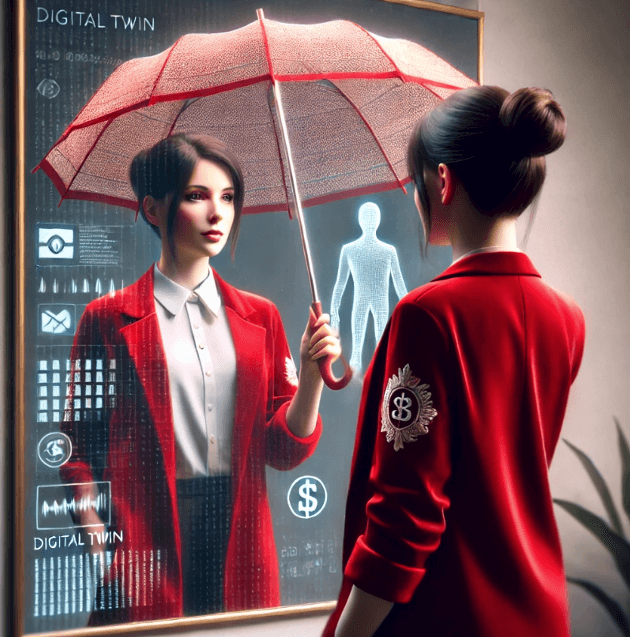
1. The Evolution of Digital Twins: Powering the Next Generation of Banking
Did you know that a technology originally designed for space missions is now revolutionizing how banks operate and serve their customers? Digital twins, first conceptualized by NASA in the 1960s for spacecraft troubleshooting, have evolved into a game-changing tool that's reshaping the banking industry. But what exactly makes this technology so transformative for financial institutions?
Digital twins are virtual replicas of physical objects, systems, processes, or people, created within a digital environment and continuously updated with real-time data. This technology has come a long way from its aerospace origins, particularly with the integration of large language models (LLMs) and artificial intelligence (AI). In banking, these virtual twins now simulate everything from customer behavior to market trends, enabling banks to optimize operations and predict future scenarios with unprecedented accuracy.
The banking sector has embraced digital twins to transform multiple aspects of their operations: from simulating market conditions and assessing risks to predicting customer needs and streamlining services. This virtual framework allows banks to test new products, optimize customer experiences, and make data-driven decisions without real-world risks. According to McKinsey research, this potential hasn't gone unnoticed, with 70% of senior technology executives at large enterprises actively investing in digital twin technology. As these virtual models continue to evolve alongside AI advancements, they're set to drive even greater innovation in financial services, making banking more efficient, resilient, and customer-centric than ever before.
(https://www.mckinsey.com/featured-insights/mckinsey-explainers/what-is-digital-twin-technology)
2. Types of Digital Twins in Banking
In today’s rapidly evolving banking landscape, digital twins have emerged as powerful tools across five distinct levels of complexity. These virtual replicas are transforming how banks operate, from foundational modeling to autonomous decision-making. Each type serves a unique role in the banking ecosystem:
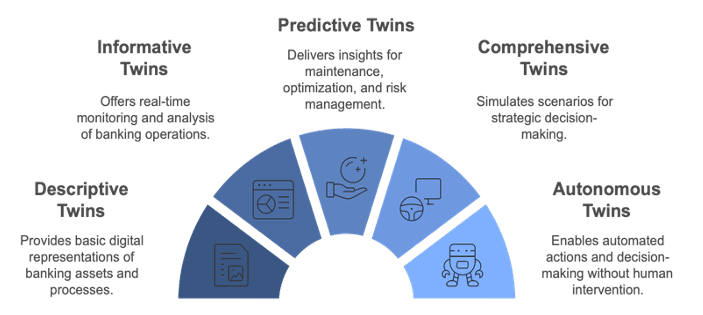
- Descriptive Twins provide live, editable versions of the bank's design and operational data, serving as a digital representation of existing systems and processes. They document basic banking processes, establishing a foundational digital model of the bank's physical assets and operations. They are blueprints of banking operations.
- Informative Twins are integrated with sensor and operational data to enable real-time performance monitoring and instant tracking of banking transactions. They offer live analysis of customer behaviors, providing valuable insights that enhance product and service performance.
- Predictive twins offer insights for maintenance and optimization through risk analysis and forecasting. They model customer behavior and predict market trends, supplying actionable information for risk management and strategic planning.
- Comprehensive twins simulate "what-if" scenarios to analyze performance under different market conditions and test possible crisis scenarios. They serve as strategic decision support systems, helping to determine optimal strategies by predicting the outcomes of potential changes or events.
- Autonomous twins can take action without human intervention by employing automatic risk management and AI-supported decision-making. They enable dynamic portfolio optimization, responding proactively to global changes and challenges on behalf of the user.
3. The Four Pillars of Digital Twins in Banking: A Comprehensive Classification
Having explored the maturity levels of digital twins and their respective capabilities, let's consider their specific applications in banking. Our analysis of digitalizable assets in the banking ecosystem reveals four fundamental classes:
- Digital Twin of Customer (DToC) simulates customer behaviors and preferences to enable personalized banking services and optimize the customer journey.
- Process Digital Twin simulates banking processes to increase operational efficiency and improve risk management.
- System Digital Twin monitors the performance of bank systems, tests security measures, and provides infrastructure optimization.
- Data Digital Twin Analyzes financial data to predict market trends and support decision-making processes.
Within the framework of these four fundamental classifications, examining the practical examples of digital twin applications in the banking sector will help us better understand the benefits and transformational potential that this technology provides.
4. Use Cases of Digital Twins in Banking
While digital twins in the banking sector provide revenue growth and operational cost optimization, they also minimize potential losses by identifying and managing risks in advance. This innovative technology, which increases customer satisfaction and loyalty through personalized services and proactive approaches, provides significant advantages to institutions in the competitive world of digital banking. Now, let's look at the use cases of digital twins in banking to understand how these benefits are implemented in practice.
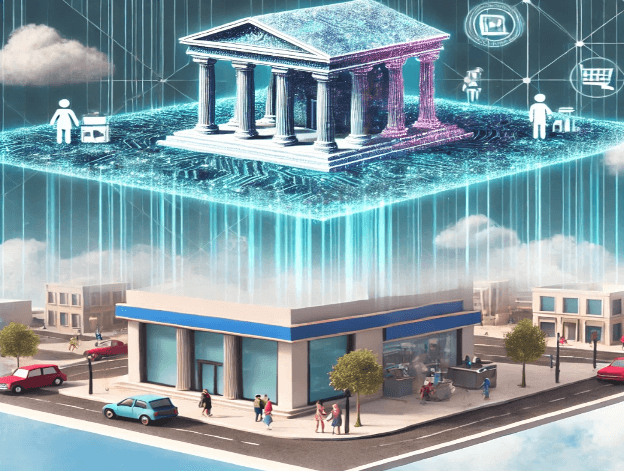
4.1 Improving Customer Experience
In the banking landscape, as in other sectors, customer experience stands out as a critical differentiator. Digital twins enable banks to understand and predict customer behavior, leading to more personalized and proactive services. This results in higher customer satisfaction, increased loyalty, and improved retention rates.
- Personalized financial product recommendations: Digital twins analyze customer spending habits, savings patterns, and life events to predict optimal financial products. This enables banks to make timely, relevant product offers that align with customer needs and financial goals
- Proactive customer services: By monitoring transaction patterns and account activities in real-time, digital twins transform reactive customer service into proactive support. This allows banks to identify and address potential issues before customers experience problems.
- Automated risk assessment: Digital twins provide continuous evaluation of customer financial health by processing multiple data points including income stability and spending patterns. This enables faster, more accurate lending decisions while reducing human bias.
Predicting customer needs through behavioral analytics. Digital twins examine transaction patterns to create comprehensive profiles of customer financial behavior. This deep understanding allows banks to anticipate needs and tailor services accordingly.
4.2 Risk Management and Security
In today's complex financial landscape, digital twins serve as powerful tools for identifying, analyzing, and mitigating risks while ensuring robust security measures in banking operations. By creating virtual replicas of banking systems and processes, institutions can proactively address threats and maintain regulatory compliance while strengthening their security posture in an increasingly challenging digital environment
- Fraud detection and prevention: Digital twins monitor individual customer behavior patterns and flag suspicious activities that deviate from established norms. This enables real-time fraud prevention while minimizing false positives.
- Credit risk analysis: By simulating various economic scenarios, digital twins evaluate potential impacts on customer loan repayment abilities. This enables banks to make more informed lending decisions and optimize portfolio management.
- Simulations against cybersecurity threats: Digital twins create virtual testing environments that replicate banking systems, allowing banks to simulate cyber attacks and test defense mechanisms without risking actual systems.
- Compliance management: Digital twins automatically monitor banking operations against regulatory requirements, identifying potential violations before they occur and maintaining detailed compliance records.
4.3 Operational Efficiency
Operational efficiency directly impacts a bank's profitability and service quality. Digital twins help optimize various operational aspects for better performance
- Optimization of branch operations: Digital twins analyze customer flow and service demands to optimize staff placement and resource allocation, resulting in reduced wait times and improved service efficiency
- ATM network management: By analyzing usage patterns and performance indicators, digital twins optimize cash replenishment schedules and prevent service interruptions across ATM networks.
- Staff scheduling: Digital twins predict customer volume and service demands to determine optimal staffing levels, helping banks maintain service quality while controlling labor costs
- Resource allocation: Digital twins process data about equipment usage and service demands to guide resource distribution, ensuring optimal placement of banking resources across the network.
4.4 Product Development
Successful product development is essential for maintaining competitiveness and meeting customer needs. Digital twins enable banks to test and refine products before launch.
- Testing of new financial products: Digital twins simulate market conditions and customer responses to new financial offerings, allowing banks to refine products before launch and improve success rates
- Measuring market response: Through sophisticated simulations of product variations, digital twins help banks predict customer adoption rates and optimize features before full deployment.
- Monitoring product performance: Digital twins track real-time usage patterns and success metrics, enabling quick identification of improvement opportunities and maintenance of product quality
- Improvements based on customer feedback: By analyzing customer interactions across banking channels, digital twins identify pain points and preferences, enabling continuous product refinement based on actual customer needs
5. Digital Twin and Emerging Technologies: LLMs, Multi-Agent Systems, and Knowledge Graphs
Let us show you how we have developed a solution using digital twin technology to enhance operational efficiency and customer satisfaction in the banking sector. By integrating Large Language Models (LLMs), Multi-Agent Systems, and Knowledge Graphs, we aim to build digital twins that are more intelligent, responsive, and data-driven. Below, we’ll illustrate how each of these technologies plays a role in advancing digital twin applications within banking.
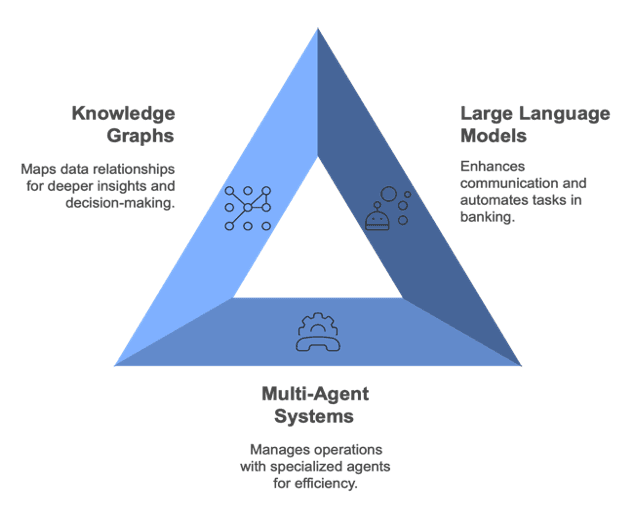
5.1 Digital Twin with Large Language Models (LLMs)
LLMs enhance the digital twin's ability to process and generate human-like language, improving interactions and automating various tasks.
- Understanding Customer Queries and Requests: Accurately interprets and responds to customer inquiries.
- Automatic Analysis of Financial Documents: Processes and analyzes financial documents swiftly without manual intervention.
- Contract and Policy Review: Efficiently examines contracts and policies to identify key terms and compliance issues.
- Sentiment Analysis and Customer Feedback Evaluation: Gauges customer satisfaction levels and identifies areas for improvement through feedback analysis.
- Personalized Financial Advice: Delivers tailored financial recommendations based on individual customer profiles.
- Automatic Report Generation: Creates detailed financial reports automatically, saving time and reducing errors.
- Customer Communication Texts: Generates personalized messages to enhance customer engagement.
- Product Descriptions and Customizations: Crafts customized product information to improve appeal and relevance to customers.
5.2 Digital Twin with Multi-Agent Systems
Multi-agent systems introduce specialized agents that perform distinct functions, enabling dynamic and intelligent management of banking operations.
- Risk Assessment Agents: Evaluate potential risks in financial operations to enhance security and compliance.
- Customer Service Agents: Interact with customers to address inquiries and provide efficient support.
- Transaction Monitoring Agents: Oversee financial transactions in real-time to detect any anomalies.
- Fraud Detection Agents: Identify and prevent fraudulent activities within the banking system.
- Coordination Between Different Departments: Enhances collaboration across departments for streamlined decision-making processes.
- Automatic Escalation Mechanisms: Automatically escalate issues to appropriate levels when necessary.
- Dynamic Resource Allocation: Adjusts resource distribution in real-time based on current needs and priorities.
- Detection of Cross-Selling Opportunities: Identifies opportunities to offer additional products or services to existing customers.
5.3 Digital Twin with Knowledge Graphs
Knowledge Graphs enrich the digital twin by mapping and analyzing complex data relationships, providing deeper insights, and supporting informed decision-making.
- Mapping Customer Relationships: Creates detailed maps of customer interactions to better understand their networks and influence.
- Product Linkages: Connects related products to identify cross-selling and up-selling opportunities.
- Risk Factor Network: Maps out how different risk factors are interconnected within the organization.
- Regulatory Requirements: Integrates and tracks compliance obligations to ensure adherence to all regulatory standards.
- Complex Relationship Analysis: Analyzes intricate data relationships to extract meaningful insights.
- Pattern Detection: Identifies recurring patterns in data that may indicate trends or potential issues.
- Anomaly Detection: Detects deviations from normal patterns that could signal problems or opportunities
- Predictive Modeling: Uses historical data to forecast future outcomes and support better decision-making.
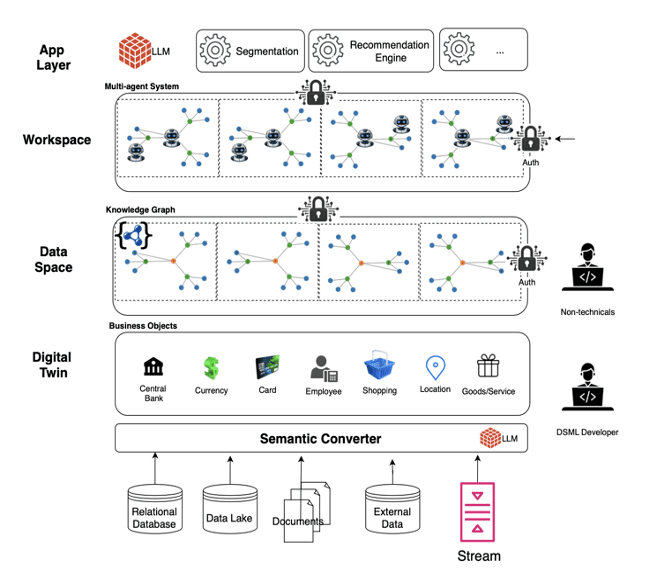
6. Advanced Use Cases of Digital Twins in Banking
To illustrate the practical applications of digital twins enhanced by LLMs, Multi-Agent Systems, and Knowledge Graphs, consider the following advanced use cases:
6.1 Credit Risk Assessment
Digital Twins Analyze Financial Documents Using LLMs: The digital twin processes and interprets complex financial statements and credit reports swiftly, providing accurate assessments.
- Multi-Agent Systems Evaluate Risk Factors Within the Digital Twin: Specialized agents within the digital twin assess various risk elements like market volatility, borrower history, and economic indicators.
- Knowledge Graphs Map Credit Histories and Relationships: The digital twin utilizes knowledge graphs to visualize connections between entities, identifying potential risk patterns and correlations.
6.2 Customer Service Optimization
- LLM-Enhanced Digital Twins Provide Natural Language Interaction: Digital twins interact with customers through conversational AI, offering intuitive and personalized support 24/7.
- Agents Coordinate Customer Requests in the Digital Twin Environment: Multi-agent systems within the digital twin manage and prioritize inquiries for efficient resolution.
- Knowledge Graphs Analyze Customer Data Relationally: The digital twin leverages knowledge graphs to understand customer preferences and history, enabling tailored services.
6.3 Fraud Detection
- Digital Twins Detect Suspicious Activity Patterns Using LLMs: The digital twin identifies anomalies in transaction data that may indicate fraudulent activities.
- Multi-Agent Systems Monitor Fraud Scenarios Within the Digital Twin: Agents continuously oversee multiple channels and touchpoints in the digital twin to detect potential threats.
- Knowledge Graphs Identify Relational Fraud Patterns: The digital twin uncovers hidden connections between fraudulent activities and entities through relational analysis.
By integrating these advanced technologies into digital twins, banks can significantly enhance their operational capabilities. This unified approach enables smarter decision-making, strengthens security measures, and delivers more personalized services to customers.
7. Conclusion
Digital twins are key to unlocking real value from LLMs and other AI solutions. While many organizations today are working to integrate AI capabilities into their business processes, without a structured and methodological approach, these initiatives often fail to extend beyond simple chatbot applications. At this stage, Twinize offers not only a technological platform but also the methodological framework that organizations need for their intelligent digital transformation journey. By taking a holistic approach that integrates data, processes, and human elements, Twinize guides organizations in effectively utilizing AI technologies to create real business value. Through this comprehensive approach, Twinize serves as a trusted partner in AI transformation, enabling organizations to achieve sustainable and scalable success while optimizing data-driven decision-making processes.